Feedback Loops
A case for optimism about AI, and some experiments that give me hope
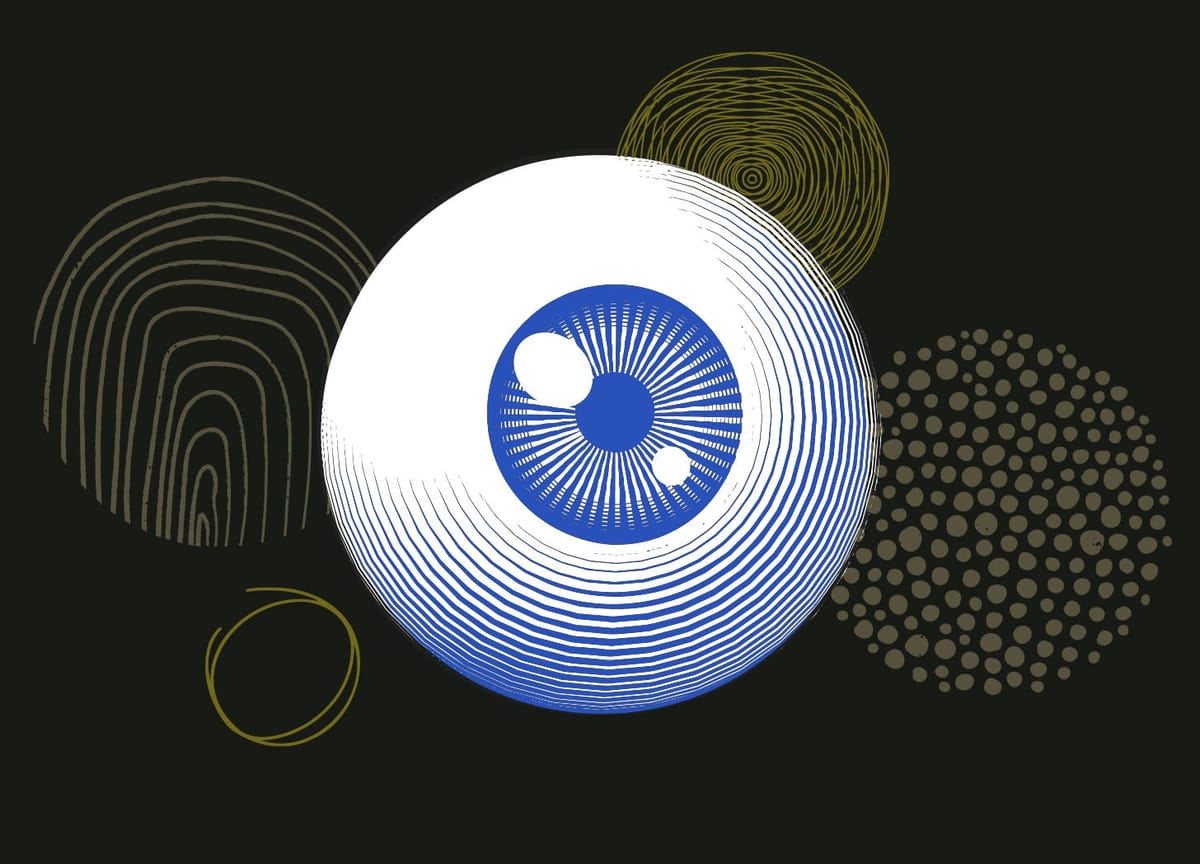
Hi everyone,
Lord have mercy on my soul, for I am about to add to the AI discourse. I spent much of 2022 dipping my toes into conversations around generative AI, not least because of the popularity of tools like DALL·E 2 and Midjourney. But in November, those conversations accelerated with OpenAI’s release of ChatGPT. The tool didn’t represent a new technology—GPT-3, the large language model (LLM) it is based on, was released in summer 2020—but rather a new interface, which made interacting with the AI like texting a friend. What had been the province of academics and extreme early adopters was suddenly legible to your parents. And it’s likely they signed up, too: as was breathlessly reported a month ago, ChatGPT reached one hundred million users in record time.
So of course the largest companies raced to begin sharing their versions of the product. And suddenly, in the corners of the internet where I spend a lot of my time, it was take after take after take … after take. Now that the robots were not only coming for those with manufacturing jobs, but also for white-collar knowledge workers, including writers like myself, it seemed people felt it critical to form opinions about the technology and its future—and by extension about our future.
As is often the case in discussions of novel technologies, opinions drifted toward the ends of the spectrum. On the one hand are those who see blue-sky economic possibility to be gained from AI-derived efficiencies and want to disrupt as many industries as possible. On the other hand are those who imagine ways to disrupt the semiconductor supply chain, instead, to stop the march of the super-intelligent machines bent on destroying us.
It may not surprise you to learn that, after all this reading and a little bit of tinkering, I come out somewhere in the middle—though leaning toward the side of optimism. I’m excited about what’s to come; despite being a writer and editor, I’m not worried about my livelihood. (If you really think I should be, please leave a comment or reply to this email so I can reconsider—and potentially start preparing—accordingly.) To me, LLMs like GPT-3, in their current incarnations, are a tool and a toy, not a source of terror. And like Rohit Krishnan and others, I am confident AI safety, which is often described as “alignment,” will come through market pressure and regulation.
The question becomes: what can we do with the tools now? How can we shape their strengths to boost our creativity, to help us when we are stuck, and to take away a little bit of drudgery? (My “hack,” when editing, is having ChatGPT reorganize disorderly bibliographies to match the Chicago Manual of Style’s rules.) How can we demonstrate, for ourselves and others, that generative AI should not only serve as an ever-more efficient attention vacuum for monopolistic digital-advertising businesses?
Here are a few uses that have inspired me:
- Geoffrey Litt on ChatGPT as a muse that can prompt him: “Maybe it’s more useful to think of the LLM in this case as a supercharged Oblique Strategies deck: a simple tool that draws random connections and makes it easier to keep going”
- Linus Lee has built fascinating mini personal tools: graphical interfaces for changing the length or tone of a piece of writing, for example, or to quickly focus upon the most important sentences in something he’s reading
- Dan Shipper fed years of his journal entries into GPT-3 so it could find patterns in his thoughts and behaviors, and so he could ask it questions about himself
- Ian Bicking built a fantasy world through ChatGPT and Midjourney. “I enjoy game worlds where the world seems alive and the details suggest a backstory. […] Is it possible to make a big world that is both eclectic and consistent?” (Thanks for the heads-up, Tom.)
- My colleagues at Frontier who, when facilitating a workshop, used Midjourney to instantly give visual expression to the future scenarios described by participants, both delighting them and reinforcing that their aspirations can become realities
More prosaically, I’ve admired those who have used ChatGPT for basic language-learning exercises; teachers who have created material using an LLM and then had their students critique it; or programmers who have used LLMs to automatically summarize the changes they’ve made to code, thereby saving time when submitting it for consideration.
In each case, these people are recognizing both the power and the limits of tools like ChatGPT and turning them toward creative, useful ends. Shipper, who has created half a dozen tools like his diary summarizer, puts it succinctly: “If you’ve ever been interested in tinkering or building things, now is one of the coolest times in history to do it.” His next sentence cautions that you won’t build a billion-dollar business. But not many of us need, or even want, that. What can you—what can we—do today to demonstrate the value these tools have outside the realm of rearranging the search-engine landscape?
In this newsletter a few weeks ago, I wrote about Wikipedia. Is ChatGPT today where Wikipedia was circa 2005? LLMs today and early-years Wikipedia shared a key characteristic: they are often incorrect, and the errors are in some way reflective of us. In that essay I marveled at what Wikipedia has become: inexhaustibly broad and broadly accurate. It offers a useful service to billions of people and is a testament to the power of incremental correctness.
Both Wikipedia and ChatGPT/DALL·E 2 maker OpenAI are run by nonprofit foundations. The difference between the two is that the former persists on an average donation of $15 and the latter just received $10 billion from Microsoft. To ensure the AI tools like ChatGPT become a public good, just like the former, we’ll have to be vigilant—and we’ll have to keep playing, keep discovering more ways they can serve us, then promoting them. I’m excited to see how these powerful tools get nudged in that direction and what they’ll allow us to do as they evolve.
Love all ways,
Brian
PS—One more thing: the machine intelligence displayed by LLMs like ChatGPT is often mistaken for being “human-like.” So I appreciated Vaughn Tan’s recent essay “what makes us human (for now)”: it’s “our ability to do things which are not-yet-understood, which require us to be able to create meaning where there wasn’t meaning before. The meaning of ‘meaning’ here is specific: Deciding or recognizing that a thing or action or idea has (or lacks) value, that it is worth (or not worth) pursuing.”
PPS—In January, I wrote briefly about Shopify’s anti-meeting “calendar purge.” Now Elizabeth Ayer, who works in government technology, has written a measured, precise defense of meetings that recognizes their role in intellectual work. Substitute the word creative for the word tech here: “The tech industry suffers from a deep association of work with individual productive toil, and that just isn’t what knowledge work is. In addition to being social, knowledge work is also uncertain and messy.” Next time someone spreads the gospel of “maker time” and humblebrags about an empty calendar, send them this essay.
Good Links
- 🧤 Smart gloves that translate sign language into audible speech
- 🎨 From 2013: Jennifer L. Roberts on deceleration and attention: “How can there possibly be three hours’ worth of things to see and think about in a single work of art?”
- 📸 Drew Austin: “We could update [Max Weber] for the social media age by saying that past generations wanted to be famous, but we are forced to be.”
- 💸 The Library of Economic Possibility aims “to foster a more participatory and informed culture of debate around how to design the next economy.”
- 🏥 Patricia Lockwood, with writing as entertaining as ever, on the odyssey of her husband’s intestinal obstruction: “In the cab, Jason was on all fours like a horse trying to give birth. If I concentrated, I could almost see the hoof emerging from him.”